
By Bernardo Tinti – Measurement & Impact Lead at Winclap
This article is part of the Marketing Efficiencies Playbook, a joint effort from Winclap and Softbank Latin America Fund to bring experts’ knowledge to leading startups and marketers to increase their efficiency and transform how they grow.
The challenge of Incremental CAC
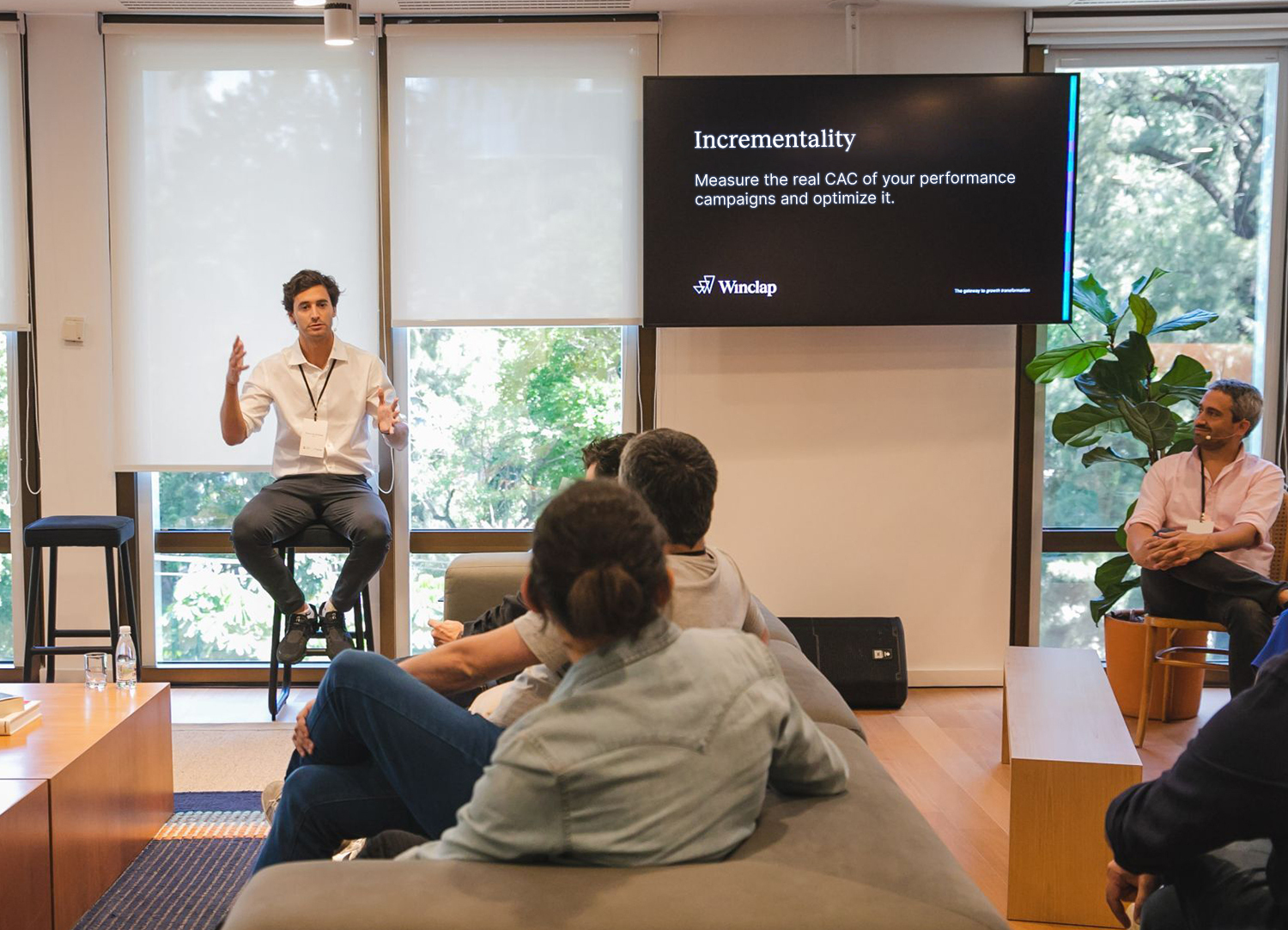
Pedro de Arteaga, COO and VP USA (left), Gonzalo Varela, VP of Growth at Winclap (right) at the Marketing Efficiency Workshop Sao Paulo edition, discussing with leading startups in Brazil how to diversify paid media effectively.
Incrementality
Incrementality refers to the true incremental contribution of any marketing action or campaign to the business outcomes they are targeting (users, events, revenue, etc). This “true incremental contribution” can be defined in opposition to the widely used “attributed contribution”.
Performance marketing teams are used to analyze campaign results and make decisions based on attribution models, which attribute events or installs to different channels based on time proximity between events and user interactions (impressions/clicks) with each channel. The usual “last touch attribution” (LTA) framework implicitly assumes that events correlated in time are also causally linked (for example, if a user sees an ad and an hour later downloads the app which the ad referred to, the attribution model assumes that the ad was the cause of the install).
But… Would the user have done the event or downloaded the app anyway if he or she hadn’t seen the ad? Incrementality measurement tries to answer this question by applying a more robust causality framework and not just mere time-based correlation (as in attribution models).
With the help of experiments and counterfactual analysis, incrementality measurement can estimate how many of the attributed events were truly (causally!) driven by each campaign, and determine the real cost of incremental events (incremental CPAs or iCPAs).
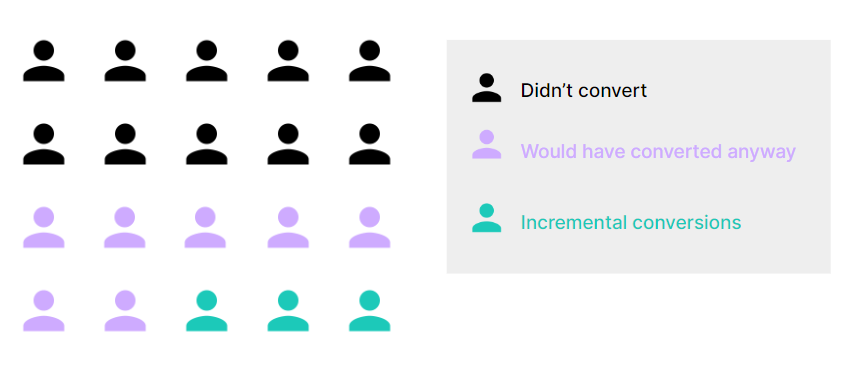
Why is incrementality measurement important?
Incrementality measurement provides a better understanding of marketing actions’ true costs and benefits, therefore allowing for more accurate and efficient marketing strategy decisions. On the contrary, the attribution models widely used by most Analytics tools or MMPs (Mobile Measurement Partners) present a series of flaws that may distort the true causal links between actions and results, and lead to suboptimal decision-making.
First, medias that show ads to users who decided to engage with an app/web by reasons other than paid media advertisements (such as word-of-mouth effects, referral programs, branding, etc) will get credit for bringing more users than they actually did. The CPA attributed to those medias (by dividing total spending over attributed events) will thus be much lower than their incremental CPA (which results from dividing total spending over incremental or causally-driven events). As a result, marketers who decide their budget allocations by minimizing attributed CPA will tend to overspend in medias with results inflated in this way.
Second, when the same users are targeted by many media at the same time, only the media which showed the last ad in which the user clicked before converting gets credit for that, although the user’s decision may have been driven by a previous ad (or maybe by none of them). As the overlap between media increases, it becomes less clear which of them is the most efficient. Even if “multi-touch attribution” (MTA) models recognize this issue and try to address it, it’s usually harder to get clear actionable from their results (which are still flawed by correlation).
All in all, decisions based on attributed CPAs often lead to paying for events that would have happened anyway, or paying twice (and even thrice or more) for the same event. The figure below summarizes how attributed CPAs may bias decision making and lead to allocate larger budgets to medias with higher incremental CPAs.
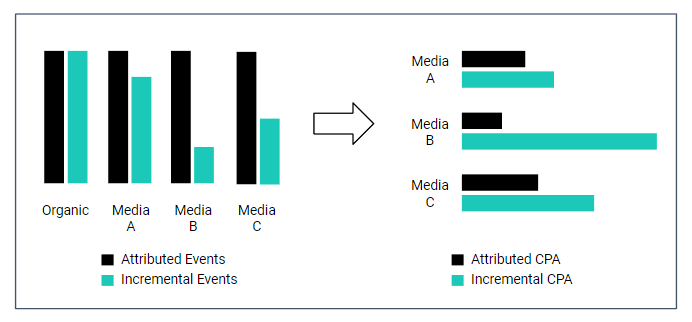
These biased decisions harm growth strategies by driving overall costs of acquisition (CAC) to levels much higher than they should, scarce budgets being wasted, and user base growing slower than it could. Incrementality-based decision making is therefore critical for startups that need to become more efficient to sustain their growth process.
Shouldn’t everyone be doing it?
Although incremental CPAs should be the key metric to analyze when deciding marketing budget allocations, measuring incrementality is not a “plug-and-play” solution, and it presents a series of challenges in its implementation. Some of them can be summarized in the following items:
- Incrementality is not usually measured on a real time or daily basis. It is measured through specific experiments.
- There isn’t a unique nor standardized framework for developing incrementality measurement experiments. A specific framework should be chosen in each situation, based on the kind of media or action analyzed, the audience segmentation possibilities, the analytical tools available, etc.
- Comparability across experiments is not straightforward. Experiments must be carefully planned, executed and analyzed for them to be comparable.
- Making decisions based on incrementality often implies a mindset transformation across the whole marketing team.
How – best practices, mistakes to avoid, Step by step
The challenges for incrementality measurement may seem overwhelming, but that shouldn’t stop you from starting your incrementality measurement journey. Depending on your company’s growth stage, there are different guidelines you may follow to improve your decision making. Here is a simple questionnaire to determine your maturity level
Have you adjusted your MMPs attribution windows? Yes / No.
If No -> Go to Basic Level.
If Yes -> Have you ever run an incrementality test? Yes / No.
If No -> Go to Intermediate Level.
If Yes -> Go to Advanced Level.
- Basic Level
The first step in attribution optimization is adjusting the click and impression attribution windows for them to capture incremental results more accurately. The windows that media and common attribution models set by default are usually very long and tend to attribute more events than those caused by each media. On the other hand, shorter windows make the total attributed events more similar to the incremental ones.
- Intermediate Level
After you have calibrated your attribution and windows and got used to running campaigns in two or three different medias, you will probably want to check if the medias you are running bring incremental results (this means, if they are really driving user behavior or if they are just attributing themselves things that would have happened anyway). The right way to do this is by executing incrementality tests.
Although there are many experimental frameworks to run these tests (ghost bidding, PSA, intent-to-treat, geo testing, etc), their main idea is basically the same. Incrementality tests compare conversion rates between a test group (exposed to advertising) and a control group (not exposed to advertising), in order to assess the causal effect of the campaigns ran in the media versus the counterfactual alternative of not running campaigns in that media. The incrementality tests’ outputs are relative uplift values (measured in different time windows) that tell us how much of the total conversions brought by the media were truly incremental. These relative uplifts allow us to detect saturated or overlapped medias, calibrate attribution models in order to show incremental CPAs, and improve budget allocations based on this information.
- Advanced Level
As your team gains experience in running and analyzing incrementality tests, and you reach a maturity level where media overlapping and saturation become a major concern, your marketing strategy should become more incrementality-driven. This means that Incrementality testing would be internalized as a usual tasks, and all the medias you’re running would be tested every 3 or 6 months. You may even have “always on” control groups (user segments, devices, geos, etc) to scale your experimental capacity, and incrementality measurement could be extended to every marketing action (including owned media, branding, discounts campaigns, etc).
In addition, being able to segment incrementality by some user signal (e.g. city) can help you optimize even further your incremental CAC. As an example, we worked with a company that decreased their iCPA by 9pp by calculating different incrementality coefficients by city (the app was widely known in the capital city of the country and its surroundings, but not in another cities in the country), and then start sending that fixed number to the medias as a value of the event, we then switched optimizations from event based, to value based. As the final step, we worked together with the company to create a LTV prediction model, which was also sent to the media to optimize, but we continued multiplying it by the incrementality coefficient, getting not only more incremental users, but also higher value ones. For more information on optimizing towards LTV, see the corresponding Playbook in Softbank’s Notion.
Finally, you can also develop Marketing Mix Models (MMM) and calibrate them with the results of your incrementality tests in order to have a practical tool for long-term marketing strategy analysis and design, as well as including campaigns that are not currently tracked in your attribution model (offline campaigns, campaigns that don’t drive clicks to your website/app, etc).
MMMs are statistical models designed to measure the impact of several marketing inputs on a certain outcome (users, conversions, sales, etc). The purpose of using MMMs is to understand how much each marketing input (paid media, branding, offline campaigns, etc) contributes to the overall result. Although MMMs rely on usual linear or nonlinear regression models (and therefore they don’t show incrementality by themselves), their parameters can be calibrated with the results of incrementality tests for them to internalize proven causal relations. For example, Meta’s Robyn open source MMM platform allows to include incremental events estimated through experiments as an input variable for optimization. You can contact Winclap if you want to learn more about using MMMs to redefine your marketing strategy.
If you are interested in optimizing your growth strategy through incrementality measurment, a reliable growth partner like Winclap can help you by:
- Develop your customized incrementality roadmap, step by step.
- Testing methodologies adapted to each particular situation.
- Plan, coordinate and execute incrementality in a consistent and comparable way.
- Attribution model calibration based on test results.
- Outsourcing and internal capabilities building at the same time.
Please feel free to contact us!
Ready to transform how you grow?
Recent Comments