In today’s marketing landscape, with advertising inventory proliferating across multiple channels, maximizing returns has become a complex, multidimensional problem. Scaling digital products at double-digit growth rates requires testing and optimizing thousands of ad campaigns on multiple channels simultaneously, each of them with its own saturation curve. This means that for every campaign, marketers face a critical decision: Spend too little and hurt revenue, spend too much and affect profitability.
The problem looks like this:
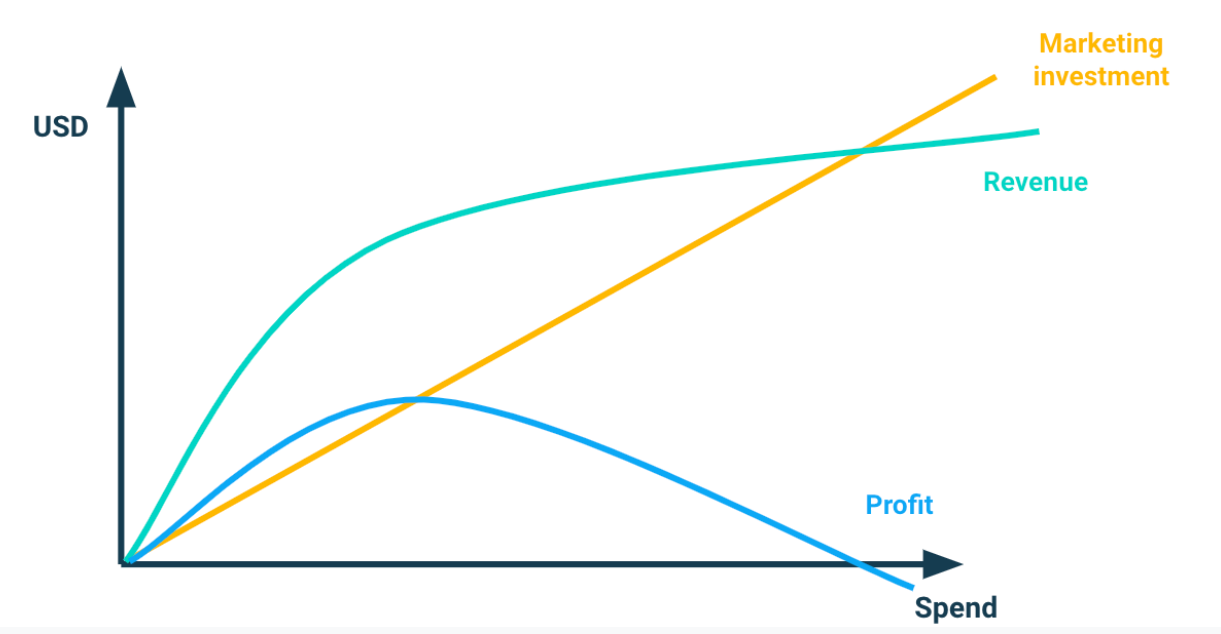
Every marketer encounters this issue: how much budget should I allocate to each campaign? Budget allocation decisions are increasingly complex if they need to be made on different levels (ad group, campaign, GEO, Media Source, app), which makes having the right tools to make the decision more important.
We’ve come to notice that many app developers still do not find this to be a critical issue, even when the industry has been disrupted by the introduction of Apple’s new privacy guidelines. Thankfully, these are not the majority, and we think Apple’s privacy-oriented policies have only increased the necessity of AI to make better budget allocation decisions, for the following reasons:
- Marketers already need to have a multi-channel approach to achieve optimal performance. Since the pool of app install ad spaces is shrinking by the hour, costs are increasing and more channels need to be considered in the media mix. Here you can find a great post about this by Thomas Petit.
- Managing multiple channels without the right tech stack to make high-quality decisions is not cost-effective. With the right tools, these decisions will be more accurate and will happen faster.
- ROAS will be affected differently in each Media Source, according to how quickly they adapt their tech to the new landscape. Most products will need to make quick budget allocation decisions to achieve performance KPIs.
Also, I believe the most important reason app developers need AI to make better decisions is that there is a significant upside in performance. We are all performance-oriented marketers and we tend to maintain budgets for long periods of time when a campaign is stable and reaches our ROAS (or CPA) target predictably. By doing this, we face high opportunity costs: those budgets could go to a better performing campaign on a different channel that we haven’t tested yet (just thinking about this can get you through an unending rabbit hole of anxiety and desperation caused by FOMO). And to add to that anxiety, we’ve found that this is true in most cases. 🙂
Since we launched our Budget Allocation tool internally, we’ve recommended changes for up to 30% of historical campaign budgets and we’ve increased ROAS by more than 25%. This means that we have reallocated up to 30% of the budget from good performing campaigns to better performing campaigns, increasing ROAS by 25% as a result. We’ve posted a more technical post here if you are interested in how the model works.
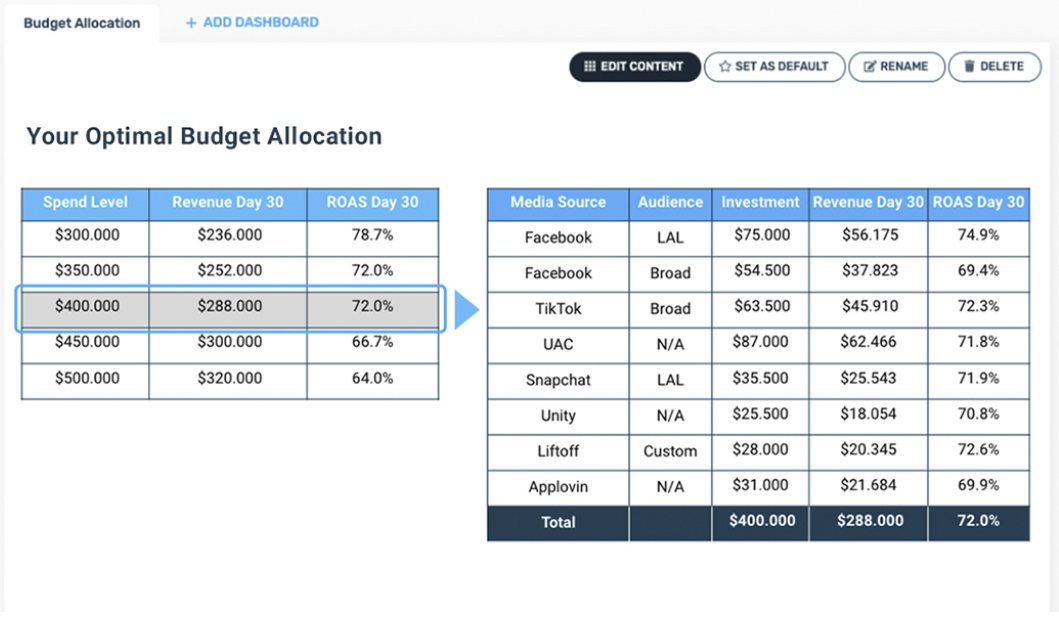
One of our early adopters is MercadoPago by Mercado Libre is the largest fintech in Latin America and have super-aggressive growth goals. We helped them in reducing CPA’s, maintaining multi-million dollar monthly spending. After a quick onboarding and fine-tuning on our AI, we started pushing budget recommendations on a weekly basis. Within the first 2 weeks, we decreased CPA by 22% for one of the GEOs and close to 10% for the other.
Actually, we are now using the tool to explore new spend levels based on the model predictions. This is an interesting use case for companies that are finding saturation in the traditional channels or companies that are trying to unlock new growth opportunities. With our AI, they can accurately predict how much revenue they’ll get for each of the new media sources they test, and allocate budget accordingly.
AI has promised a lot to advertisers all over the world, and in many ways, it has fulfilled that promise. It has done a great job in automating narrowed-down actions but hasn’t kept its promise in helping in high-level decisions — the basis of all other decisions — like how to allocate budgets in multiple campaigns, which creative messaging will perform better, and how to bid accordingly, among others. All these decisions are currently made based on some data and a lot of intuition, and this sort of works but has tremendous potential of being improved. That is what we’re trying to solve here at Winclap.
We are looking forward to seeing where this takes us, and to helping more and more digital products to scale sustainably.
Recent Comments